共计 2455 个字符,预计需要花费 7 分钟才能阅读完成。
tf.ConfigProto()函数用在创建session的时候,用来对session进行参数配置:
config = tf.ConfigProto(allow_soft_placement=True)
config.gpu_options.per_process_gpu_memory_fraction = 0.4 #占用40%显存
sess = tf.Session(config=config)
- 记录设备指派情况 : tf.ConfigProto(log_device_placement=True)
设置tf.ConfigProto()中参数log_device_placement = True ,可以获取到 operations 和 Tensor 被指派到哪个设备(几号CPU或几号GPU)上运行,会在终端打印出各项操作是在哪个设备上运行的。
- 自动选择运行设备 : tf.ConfigProto(allow_soft_placement=True)
在tf中,通过命令 “with tf.device(‘/cpu:0’):”,允许手动设置操作运行的设备。如果手动设置的设备不存在或者不可用,就会导致tf程序等待或异常,为了防止这种情况,可以设置tf.ConfigProto()中参数allow_soft_placement=True,允许tf自动选择一个存在并且可用的设备来运行操作。
- 限制GPU资源使用:
为了加快运行效率,TensorFlow在初始化时会尝试分配所有可用的GPU显存资源给自己,这在多人使用的服务器上工作就会导致GPU占用,别人无法使用GPU工作的情况。
tf提供了两种控制GPU资源使用的方法,一是让TensorFlow在运行过程中动态申请显存,需要多少就申请多少;第二种方式就是限制GPU的使用率。
一、动态申请显存
config = tf.ConfigProto()
config.gpu_options.allow_growth = True
session = tf.Session(config=config)
二、限制GPU使用率
config = tf.ConfigProto()
config.gpu_options.per_process_gpu_memory_fraction = 0.4 #占用40%显存
session = tf.Session(config=config)
或者:
gpu_options=tf.GPUOptions(per_process_gpu_memory_fraction=0.4)
config=tf.ConfigProto(gpu_options=gpu_options)
session = tf.Session(config=config)
设置使用哪块GPU
方法一、在python程序中设置:
os.environ[‘CUDA_VISIBLE_DEVICES’] = ‘0’ #使用 GPU 0 os.environ[‘CUDA_VISIBLE_DEVICES’] = ‘0,1’ # 使用 GPU 0,1
完整的config配置
allow_ |
bool allow_ |
cluster_ |
ClusterDef cluster_ |
device_ |
repeated DeviceCountEntry device_ |
device_ |
repeated string device_ |
experimental |
Experimental experimental |
gpu_ |
GPUOptions gpu_ |
graph_ |
GraphOptions graph_ |
inter_ |
int32 inter_ |
intra_ |
int32 intra_ |
isolate_ |
bool isolate_ |
log_ |
bool log_ |
operation_ |
int64 operation_ |
placement_ |
int32 placement_ |
rpc_ |
RPCOptions rpc_ |
session_ |
repeated ThreadPoolOptionProto session_ |
share_ |
bool share_ |
use_ |
bool use_ |
运行TensorFlow图时,有几种可能的并行形式,这些选项提供了一些控制多核CPU并行性:
- 如果您具有可以在内部并行化的操作,例如矩阵乘法(
tf.matmul()
)或归约(例如tf.reduce_sum()
),TensorFlow将通过在具有intra_op_parallelism_threads
线程的线程池中调度任务来执行该操作。因此,此配置选项控制单个操作的最大并行加速。请注意,如果并行运行多个操作,则这些操作将共享此线程池。 - 如果您在TensorFlow图中有许多独立的操作-因为在数据流图中它们之间没有直接的路径-TensorFlow将尝试使用带有
inter_op_parallelism_threads
线程的线程池并发运行它们。如果这些操作具有多线程实现,则它们(在大多数情况下)将共享同一线程池以进行操作内并行操作。
Q:tf.train.MonitorSession 与 tf.session 之间的差异?
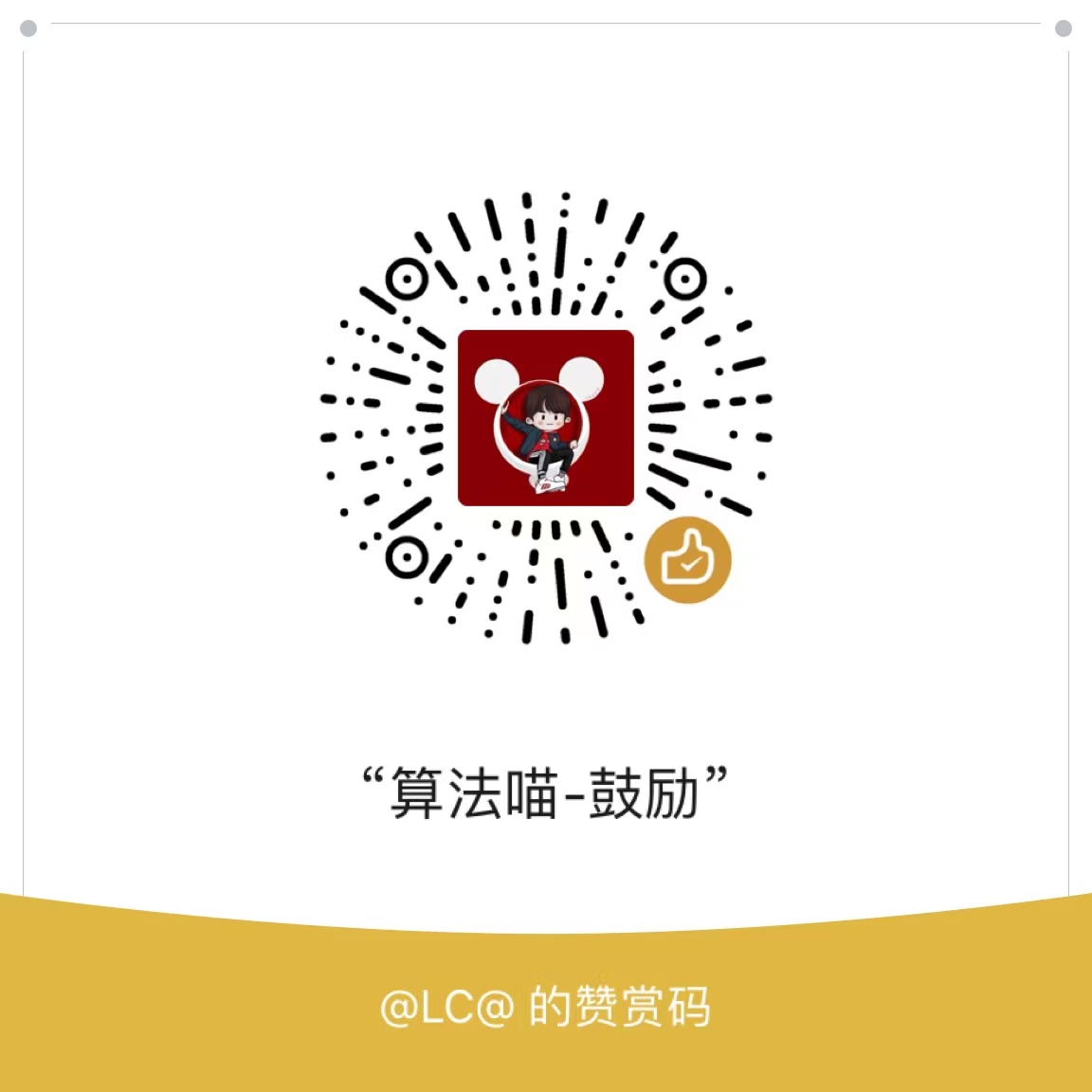